You can choose “accuracy” or “precision.” Not both.
[ This article originally appeared on my substack posting on Sept 19, 2023. I’ve been collecting feedback from readers on various platforms, and will be posting follow-ups there. You can subscribe to my newsletter there to get updates immediately. I will also repost here. ]
Introduction
When you take your pulse, whatever your reading is, well, that’s your pulse. It is what it is. Same with your blood pressure, temperature, and cholesterol levels, and almost everything else. Each of these can change, but when they do, the new reading reflects that new level. In other words, the value is systemic in your body.
In the diabetes world, to know what your blood glucose level is, you use a blood glucose monitor (BGM) or a continuous glucose monitor (CGM). And, if it reports 95 mg/dL, that reading is similar to the other biomarkers: “It is what it is.” Similarly, as your glucose level changes, each new reading reflects that new value. It’s “systemic.” Any variation from the device’s reading and the actual amount of glucose in the body is more due to an error in the device.
For this reason, if you want to optimize diabetes management, it seems reasonable to improve devices’ level of accuracy.
Enter Dexcom’s new G7 CGM and its claim of improved accuracy over the G6 (and all other CGMs). Sounds great, right? And though it may sound counter-intuitive to ask, does greater CGM accuracy actually translate to better glycemic control? If so, how? If not, why not? Or, counter-intuitively, could it make glycemic control worse?
Maybe “it is what it is” doesn’t apply to glucose levels. Maybe these readings aren’t systemic.
A sneak peek at Dexcom G7 data
When my first box of Dexcom G7’s arrived in March 2023, I was as giddy as a kid at Christmas. Accuracy notwithstanding, the G7’s other great features are to die for! (Sorry. It’s a figure of speech.) It’s 60% smaller than the G6, it’s easier to apply, its warmup time is 30 minutes (vs. 120m for the G6), there’s a 12-hour grace period for sensor expiration, and a new sensor can be started while continuing to wear the one that’s expiring, allowing the user to continue seeing data during the new sensor’s warm up. These are all big wins over the G6.
I ripped open the new box, downloaded the new G7 app (which is different/better than the G6’s), and started the first sensor. Easy and fast. In 30 minutes, the magic began.
As it happens, I was still wearing an active G6, and there was no need to turn it off because the two sensors have their own apps that could be run simultaneously. Because both send their data to the Dexcom Clarity portal, you can see both at the same time on the Clarity app. But because the app is in black and white, you can’t tell which readings go with which sensor.
A better way to view and analyze such data is to download it from the Clarity portal, and import the resulting CSV file into a spreadsheet. From there, you can create graphs, analytical formulas, tables, and so on. Below is a 24-hour graph of the G6 and G7 data side-by-side. (Note: I also loaded data from my blood glucose monitor, the Contour Next One, which connects to my phone via bluetooth, and whose data can also be downloaded from the cloud.)
The most striking and obvious quirk about is the G7 that everyone has been talking about is the jumpy glucose values. Some celebrate this as finally having what we hoped for–greater accuracy–and that it just requires getting used to. But I intuited there was probably a lot more to the story than just that. No one is asking–or even testing–the elephant in the middle of the room: Do each of these individual readings, accurate though they may be, help T1Ds make better, more informed management decisions?
Given the setup I just designed, I figured I’m set up to test exactly that.
Comparing G7 vs G6 time-in-range stats
During March, 2023, I wore both the G6 and G7 at the same time for 30 days (though I intended to do 90 days), during which time I would only observe data from one sensor’s app at a time to make real-time management decisions. After a period of a few days, I switched to the other sensor’s app, and repeated this pattern several times during the month. Upon completion of the experiment, I downloaded all my data to Excel and analyzed it to see how my TIR varied between the two. (I also collected data for insulin (InPen bluetooth enabled insulin pen), carbohydrates, exercise, sleep, and glucose levels from my Contour Next One blood glucose meter (BGM), which I included in my analysis report.)
Spoiler Alert: I intended to run the experiment for 90 days, but I had to stop at the end of one month because my performance with the G7 was so abysmally poor that it didn’t make sense to continue. Obviously, I represent only one person, so this does not say anything about others’ experiences. Nevertheless, there are critical and subtle issues revealed in my experiment that are worthy of consideration.
The graphic below is the topline dashboard from my month wearing both the G6 and G7:
The first thing that pops out is that the G7 reported glucose values ~5% lower than the G6 (consistent with what others have reported online). Aside from that, the two sensors appear roughly equivalent: The G6 averaged 121 mg/dL, versus the G7’s 116, and the standard deviations (SD) were 33 vs. 34, respectively.
But the real difference between the two sensors is shown by the time-in-range (TIR) stats on a day-by-day basis, as shown in the following graph:
When I used the G6 to make decisions, I achieved a TIR of >90%. When I used the G7, my TIR dropped to the ~70% range. And you can see this remains consistent with the periodicity in the bar chart. To understand why the G7 made it harder for more to maintain glycemic control, let’s look more closely at the earlier chart, which is a day where my decisions were governed by the G7’s data.
Now, let’s zoom into the two-hour window between 4-6pm, which is highly representative of the kind of volatility there is in G7 data versus the G6, and why it’s hard to make real-time decisions.
Remember, I couldn’t see the G6 data (the smoother blue graph), so at 5:30pm, and with only the G7 data in view, I saw the very rapid rise from 88 to 155 in a matter of 30 minutes. Granted, the data leading up to that was highly erratic, but these successive readings were not–they were decisively rising, and fast. Without any idea where these levels might top out, I knew I needed to start bolusing. As I always do, I began small and incremental boluses, keeping a close eye on those glucose levels as they rise, waiting to see when they level off or begin to fall, hoping not to take too much.
Turns out, the G7’s data shot up to 270. If this really was my real glucose level, the stacked boluses would have perfectly corrected these readings, and I would have had a soft landing. But, as the insulin started to kick in, my glucose levels plummeted to 49, making it clear to me that the G7 readings were not giving me reliable information. They may be “accurate,” but unreliable. I needed to investigate this.
In short, the G7’s readings, while potentially “accurate,” were mostly anomalous, at least insofar as using them to make management decisions. How can “accuracy” and erratic readings co-exist? Either individual glucose readings are not representative of whole body glucose levels, or systemic glucose levels are more volatile than we thought. Perhaps both.
We can see from the larger time scales that systemic glucose movements can be captured equally well by both the G6 and G7, but the problem is the small time scales: Do they offer useful enough information to make real-time management decisions? This is a technique called, “sugar surfing.” From that article,
“Sugar Surfing is the skill of making in-the-moment self-care decisions using real-time blood sugar readings displayed on a continuous glucose monitor (CGM). It involves identifying shapes and patterns in CGM trend lines that serve as a heuristic or mental shortcut to quickly process and interpret the data.”
Some find sugar surfing challenging to learn, as it requires a reasonably adept level of knowledge of glucose and insulin metabolism. Since the key is identifying shapes and patterns in CGM trend lines, that’s where the G7’s volatility makes it hard, because those patterns don’t emerge for too long of a time period to take preemptive action.
In short, it not possible to “get used to” the G7 readings if you make decisions from short time windows. And everyone that’s in tight control uses short time windows. (Most people aren’t in tight control, and typically work on bigger time windows, so they won’t be as affected by these erratic readings.) For those who wish to react in time to correct for trends, the conundrum for the G7 is that your sugars may look like they’re starting to move up/down, but then the data suddenly reverses 30 minutes later because those earlier readings were anomalous. Therefore, you can’t “react” to movements until the trend line is reliable enough. If you believe the trend too soon, you could be right, but you could also be wrong. And by then, it’s way too late. So no, you can’t get used to that. Over time, your bets will eventually reveal an upper limit on a your TIR, which is the ultimate measure of glycemic control. I would predict that the G7’s volatility will impose an upper limit on a user’s TIR.
Here are more daily charts to consider (without additional commentary). You can zoom in on your own and guess how/why I was able–or unable–to see trends in time to make decisions proactively.
The paradox seems to be that the G7’s improved accuracy resulted in worse glycemic control, at least for me, and I suspect this would be the case for others who rely on short time windows of CGM data to make in-the-moment decisions (similar to the “sugar surfing” method).
This raises the obvious question about why the G7’s accuracy is the way it is, whether it can be useful, and what we can learn from it.
The Dexcom G7 trial: Exploring the futility of “accuracy.”
In Dexcom’s published report, “Accuracy and Safety of Dexcom G7 Continuous Glucose Monitoring in Adults with Diabetes,” 318 diabetic subjects wore three G7 sensors simultaneously over the course of ten days. For three of these days, subjects underwent clinically induced hyperglycemia and hypoglycemia under controlled conditions, where blood samples were taken and measured using a reference blood glucose sensor, the YSI 2300 Stat Plus glucose analyzer. The analysis showed that the “mean absolute relative difference” (MARD) between the two was ~8.8% for the G7, versus ~10% for the G6. The lower the percentage, the smaller the difference to the reference analyzer. Hence, greater accuracy.
While Dexcom’s trial showed that the G7’s MARD values were good–in fact, better than all other CGMs on the market–the devil is in the details. Those MARD values varied considerably under different conditions, as shown in this figure from their report.
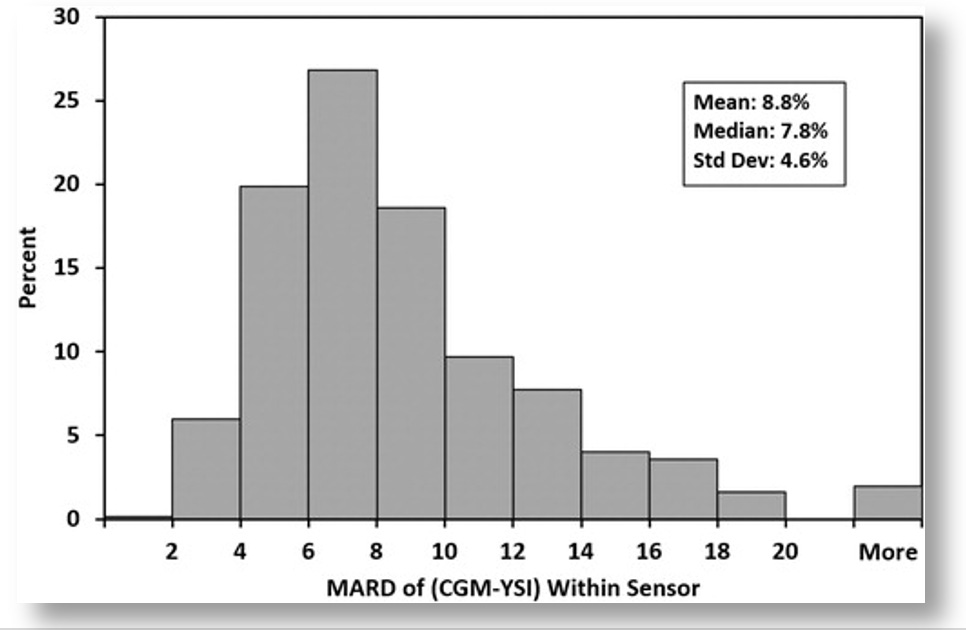
The bar graph only shows the percent of sensors within each MARD level, but not the glucose values associated with each threshold. To get that, we can look at other tables in the report, which show how the G7’s accuracy correlated with different glucose values. In short, the accuracy was best when glucose values were in the sweet spot of glycemic ranges, but accuracy diminished at more extreme glucose levels.
Remember, the trial only aimed to measure accuracy, and it put subjects under specific glycemic levels by infusing them with insulin and glucose. Accordingly, the trial revealed that the G7’s level of accuracy varied by glucose levels. But T1Ds don’t spend their lives under controlled conditions at set glucose levels. In the real world, they’re all over the map. Now that we know the accuracy rates for specific glucose levels, the next question is, how much time do T1Ds spend at each of those levels?
This metaanalysis of multiple studies on overall glucose levels for T1Ds who wear CGMs shows that only 30% of T1Ds have glucose ranges between 70-180 mg/dL 70% of the time, which is where the G7 is most accurate. By contrast, 80% of T1Ds spend more than 70% of their time above 180 md/dL, where the G7’s accuracy exceeds 30% error. (For context, 44.5% have an A1c between 7–9%, 32.5% exceed 9%, and only 23% of T1Ds had an A1c <7%.)
Despite the fact that the G7 is the most accurate sensor on the market, T1Ds are experiencing accuracy error rates of >30% most of the time.
It raises two critical questions: Why is this accuracy thing so hard to nail down? And given this poor level of accuracy, how can we rely on these readings to make good management decisions? There are three essential elements to this:
- Glucose is not evenly distributed in the body.
- Glucose is highly erratic because of diabetes.
- It’s very hard to get reliable readings from complex fluids like blood and interstitial fluids.
What we’ll find is that individual glucose measurements don’t give you very useful information, irrespective of accuracy. Even for the sake of argument that these individual readings are 100% accurate, the numbers are so erratic, what value can they possibly bring? As noted earlier, taking action too soon on any given glucose reading isn’t wise. You need to wait to ascertain a trend. The G7 makes it too hard to see trends in short time windows.
Again, what you really want are trends, which rely on a combination of accuracy and statistical probabilities. That is, what’s the range of likely glucose values that would follow the previous reading, or some number of prior readings. That’s a complicated problem to solve, so let’s go through the three bullet points.
Glucose Distribution
Glucose is the primary fuel for life to exist: Muscles use it to move, your brain uses it to think, and literally every bodily function that requires energy must break down glucose into substrates that allow electron transfers. Hence, different places require different energy levels, which is going to see glucose move through the bloodstream to get to these target locations. It’s like traffic in a highway system: Some areas are going to be more congested than others.
The biggest consumer of glucose of all is the brain. Although it represents only 5% of total body mass, it holds about 20% of the body’s total glucose volume. That’s a huge imbalance of glucose relative to the total blood supply. Moreover, the brain metabolizes glucose at the rate of ~5.6 mg per 100g of human brain tissue per minute, so a lot of mechanisms are geared towards moving glucose to the brain.
A similar-but-different process happens with other organs and limbs as well. Muscles use glucose to generate energy, and muscles pretty much cover our entire body, but some more so than others.
There are also other organs, such as the liver, kidneys and others that utilize glucose in different ways in different quantities.
The wide distribution of glucose in all these areas of the body can be tested using a standard BGM using blood samples from fingers, forearms, upper arms, legs, and so on. There are also mysterious about why glucose resides more in one area over another. In a 2020 paper called, “Differences in glucose levels between right arm and left arm using continuous glucose monitors,” the study’s authors wrote that “glucose levels in the right arm were higher than the left arm in 67% (range 46–98%) of all time-matched readings,” independently of handedness, gender, race, age or other factors. Indeed, Dexcom’s own clinical trials (the G7 and all the way back) show these differences as well: When sensors are placed on different parts of the body, different readings are obtained, and different levels of accuracy are ascertained.
Glucose Volatility
The wide distribution of glucose is variability, but the rate of movement contributes to volatility. To be sure, this is a very erratic and imperfect process, and even non-diabetic bodies get it wrong more often than we think. A study from Stanford University showed that healthy, non-diabetic subjects wearing CGMs had TIR levels of 96% most of the time, and often showed glucose movements that were difficult to explain, appearing similar to T1Ds. True, non-diabetics rarely go far outside of normal glycemic ranges, and they do snap back again more quickly, but volatility was still far more evident than what had previously been assumed.
Measuring Glucose in Fluids: Sample Disparity
Now that we know that glucose is widely distributed, and it’s highly volatile, the next big challenge is actually trying to measure it.
Even a tiny sample of blood or interstitial fluids contains a morass of disparate molecules of different sizes and properties that form crowding effects: Cells, proteins, antibodies, DNA fragments, viral particles, and junk of all sorts interact with one another in ways that force other molecules–including glucose–into whatever space is left over within any given fluidic volume. That means that any given sample may have different concentrations of glucose molecules from the next sample.
Lab-based glucose levels that are derived from venous blood draws from the arm involve a far larger volume of blood, so the ratio of glucose to that volume is more representative of the whole body. But when sample sizes get smaller, variability between samples increases. As test strips for BGMs required progressively smaller volume requirements, variability between results increased. Same thing with interstitial fluid used by CGMs: One sample can show a reading of 95 mg/dL, and the next reading could be 100 mg/dL and the one after that 80 mg/dL. Once again, Dexcom’s published data reflects this phenomenon by virtue of the ratio of errors they report for each reading, a phenomenon that gets progressively more erratic when glucose levels fluctuate or are elevated.
Not only does this demonstrate that accuracy has its limits, its very definition isn’t what people think it is. One reading is not representative of the whole body. It only tells you much is in that sample, which will likely be different from the next sample.
All three of these factors–distribution, volatility, and sample disparity–casts a shadow on the usefulness of “accuracy” as a metric for evaluating glucose sensors and meters. What use is there to having a highly accurate glucose reading if there’s no actionable information that can be derived from it?
Instead, one needs to aggregate multiple readings to glean trends. It’s not really a matter of smoothing the data, or calculating averages from multiple reads. It’s more complicated than that. That’s what brings us to the difference between accuracy and precision.
CGMs and the “precision decision”
Accuracy refers to how close a single measurement is to a true or accepted value, whereas precision refers to how close multiple measurements agree with one another.
If the G7 is more accurate than the G6, why did I achieve better TIR ratios with the G6? Because, it turns out, “accuracy” is not where the value is–it’s precision. And the G6 used proprietary algorithms to calculate a more reliable (and realistic) pattern of glucose trends.
Using the 5:30pm time window from the earlier example, we have both G6 and G7 data to look at. The G6 had 137, 135, 133, 130, 126, 124, 121, and 118, etc., which are similar enough to one another that the trend is apparent and decisions can be made. By contrast, the G7’s “more accurate” readings were 131, 115, 106, 115, 117, 98, 94, 89, and so on. You can certainly claim “high accuracy,” with these readings, but “low precision,” because they are too disparate from one another, making them a statistically improbable sequence, if what you’re really looking to measure are systemic glucose movements.
You can improve precision by adjusting successive readings to produce more reliable trend patterns, as the G6 did, but that reduces the accuracy rating for the sensor. Or you can wait longer than five minutes to get more data, but users already think five-minute windows are too long. Alternatively, you can get both higher precision and higher accuracy by adding two or three more sensors to produce more data per unit time, or by increasing the volume of fluid being measured, but each of those that adds to the cost and complexity of the system. What to do!?
This is an example of a well-known (and studied) phenomenon called the Two-out-of-Three Theory, which is commonly expressed as “Good, Cheap, Fast. Pick Two.”
From manufacturing to restaurant management to personal daily activities to vacation rentals–and yes, glucose sensors–the two-out-of-three problem is universally disdained by anyone having to make a business out of a product or service.
It would appear that Dexcom chose higher precision for the G6, and higher accuracy for the G7. Which model yields better glucose control is up for speculation until someone actually conducts a cross-over study similar to the one I conducted on myself. If one were done, I’d speculate the G6 will likely perform better for those in tight control (like me), but everyone else (the majority) would likely find no difference between the two. Those who might claim to do better with the G7 may be under the illusion they are because of its tendency to report lower overall averages. Remember, it’s TIR that’s more important for optimizing glycemic control.
Summary
I personally suspect that few people will find the G7 helps T1Ds improve their glycemic control–at least, not because of the data it presents. However, the G7’s other features (smaller, shorter warmup time, easier to apply, etc.) are so much better than the G6, I suspect it will succeed in the market.
As for the “accuracy vs. precision” conundrum, I speculate that market forces favored “accuracy” for a number of reasons.
First, accuracy is quantifiable–it can be measured. People like and trust things that can be measured, regardless of clinical relevance. Precision could sell too, but it’s more qualitative, a concept that’s harder for consumers to understand, especially when you’re trying to differentiate from the G6. And there’s lower risk to the “better accuracy” claim because most T1Ds are not in such tight control that they will notice any difference in their glycemic control.
The second aspect of market forces is that Dexcom’s target market is moving well beyond T1Ds. There are nearly 40 million type 2 diabetics, compared to roughly 1.5 million T1Ds. That, plus a very rapidly emerging market of non-diabetics: athletes, health enthusiasts, biohackers and everyday consumers. Non-T1Ds’ glucose levels are not volatile enough for the G7’s erratic readings to bother anyone.
Of course, the downside for T1Ds is that some could actually see worse outcomes. Perhaps worse would be a perception of glycemic improvement. As noted earlier, the G7’s propensity to report lower average glucose averages (than what is actually in the bloodstream) may give people the false impression that their glycemic control has actually improved with the G7. And most won’t really question this.
That said, Lab-based A1c levels can be used as an independent check on this claim. Though A1c levels can sometimes differ from CGM estimates, that difference is pretty constant per individual. That is, the delta between A1c values and the G6’s eA1c estimates may be non-zero for some individuals, but whatever that difference, it would be constant over time. My A1c’s have always paired exactly with the G6’s data, and given that the G7 reported 5% lower averages than the G6 as both were worn, this is clearly something that others who only wear the G7 will never notice. They will believe their glucose averages are lower, even if they’re not.
If I had my wish, Dexcom would make it possible for the user to choose among a list of approved algorithms when they view their data on the G7. “Accuracy? Precision? You choose!”
I have an idea: Conduct effectiveness trials to see which algorithm actually yields improved glycemic control. That would be novel.